The importance of data quality for analytics
- Analysis for Office, Predictive Analytics, Reporting
- analytics, data management, PDCA, sap analytics cloud
- 3 min reading time
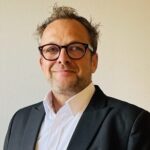
Roger Heckly
In the world of analytics, data quality is key to identifying relevant patterns and making informed decisions. Only with accurate and reliable data can companies realize the full potential of their data of their data analysis and gain valuable insights. Inferior data quality means inferior insights - a luxury no one can afford in today's data-driven landscape.
Table of contents
Why does data quality matter for analytics?

Data Driven Company or data-based decisions are approaches in which companies make decisions based on information. Such information is automatically prepared by systems based on definitions and made availablein reports or dashboards.
In doing so, one relies on the correctness, completeness, consistency and timeliness of the information. In order to trust the quality of the information , it is important that the data source is of high quality .
How is data quality defined?
Data quality refers to the accuracy, completeness, validity, consistency and relevance of data. When data is of good quality, it is reliable and can be trustworthy information.
What is meant by analytics?
Why is data quality important for analytics?
Data quality is of great importance for analytics as it has a direct impact on the quality of insights and decisions . Inthe following, some important aspects relatedto data quality are highlighted .
Analytics is based on data, and if it is flawed or incomplete, the results can be unreliable .
Good data quality ensures that the results are trustworthy and meaningful.
Analytics aims to extract accurate information. If data quality is low, errors and inaccuracies can lead to wrong conclusions .
High data quality ensures precise analysis.
Analytics is often used to support decision-making. When data quality is poor, decisions can be based on incorrect, distorted or incomplete information .
Good data quality enables informed decision making.
Data integrity is defined as the accurate and consistent maintenance of information throughout its lifetime. Data integrity also means that there is no corrupted data.
Data quality supports that relevant data is included in the analysis.
In order to gain more comprehensive insights, data from different sources are combined and harmonized in a data warehouse. Ideally, the same data also has the same types and keys.
High data quality reduces the complexity in the interfaces and the effort of harmonization and increases the quality of the analysis.
To automate processes, a system must be able to make decisions based on the underlying data. E.g. automatic triggering of a service order - whether this is created under warranty or chargeable depends on the master data in the equipment .
High-quality data is the basis for automations.
What points need to be considered when it comes to data quality?

- Completeness: The data contains all important information or values.
- Accuracy: The data is correct and precise to provide reliable results.
- Consistency: Data is consistent in terms of formats, values, and units to allow for proper analysis.
- Up-to-dateness: The data is always up to date.
- Data validation: The data are checked for plausibility and errors to ensure their quality.
- Data cleansing: The data is cleansed of inconsistencies, duplicates or error values.
- OwnerShip: It is clear in which leading system (OwnerShip) which data is maintained by whom (responsibility).
To ensure the above points, it helps tointroduce certain control mechanisms (e.g. a master data management system) as well as a process for the life cycle of an object, which clarifies the following questions, for example:
- When / why is an object created?
- In which system is it created by whom?
...
For a deeper dive into the topic, download our free whitepaper. Below you will find a brief summary of the contents:
Table of Contents Whitepaper
- Ensuring data quality through PDCA cycle
- Where do companies have the greatest deficits in terms of data quality?
- Impact of data quality in a business process
- What added value does analytics software offer me as an end user?
Webinar
SAP Datasphere & SAP Analytics Cloud feature update in Q2/24
Date: 28.05.2024
Time: 08.30 - 09.15 a.m.
Would you like to delve deeper into this topic? Then we look forward to a personal exchange on the topic of data quality for analytics!
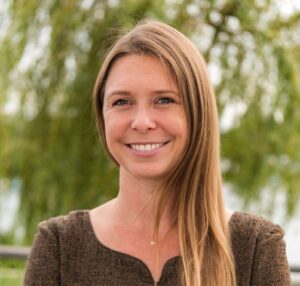
Published by:
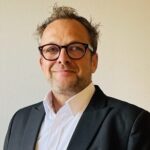
Roger Heckly
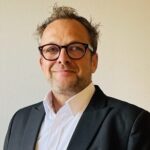
Roger Heckly
How did you like the article?
How helpful was this post?
Click on a star to rate!
Average rating 5 / 5.
Number of ratings: 4
No votes so far! Be the first person to rate this post!